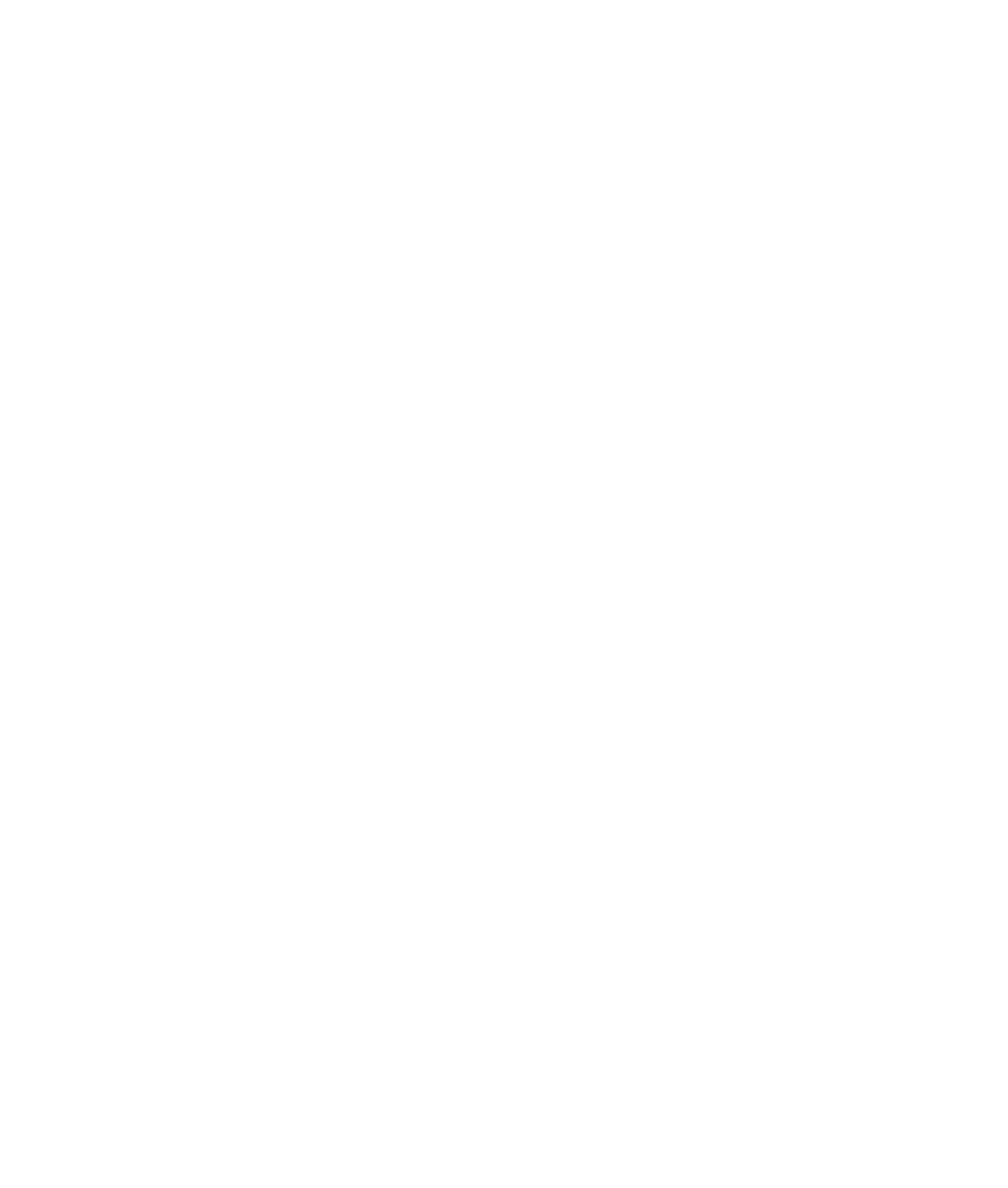
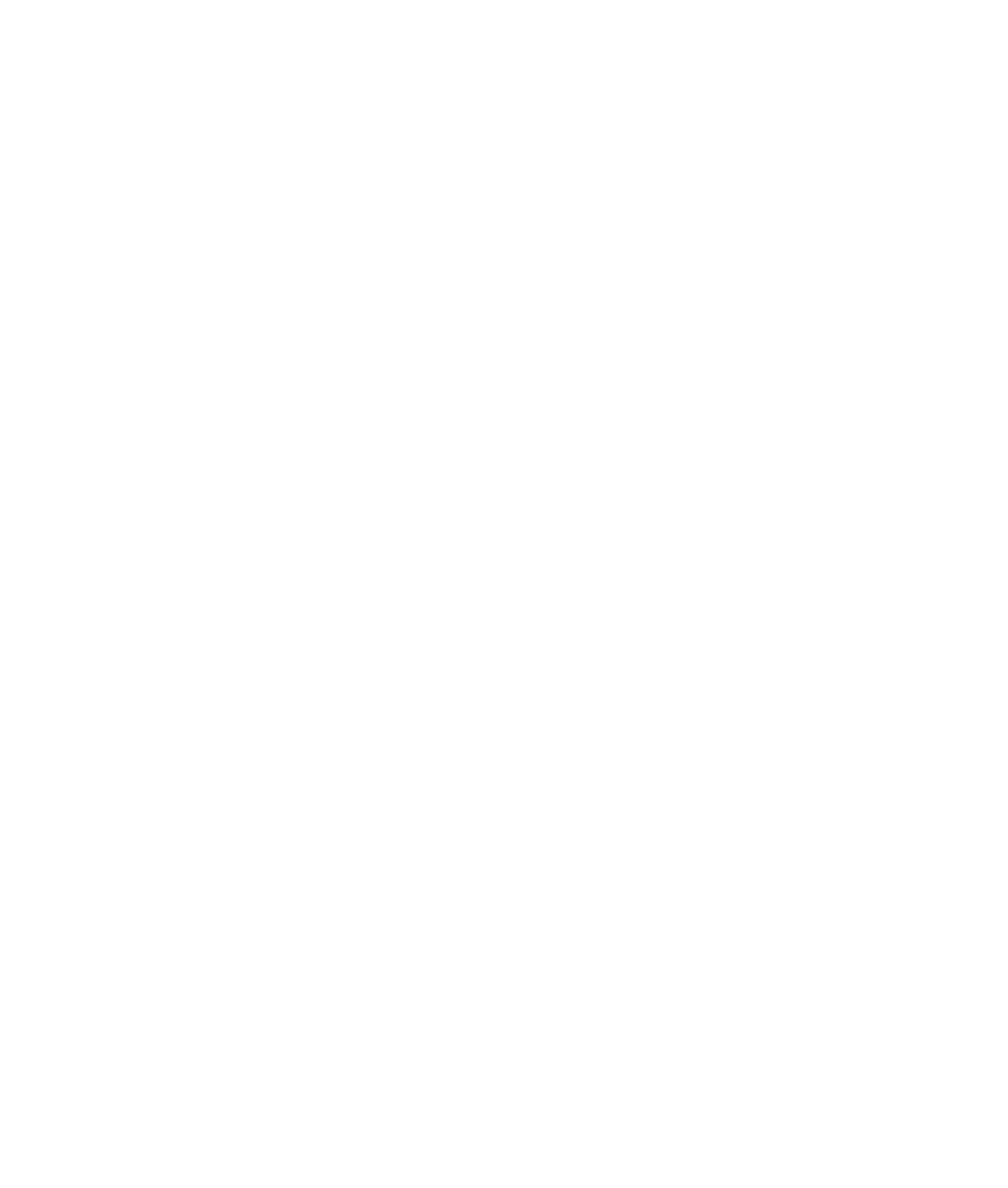
Our methodological approach
Methodology adopted
The Observatory has established a methodological approach to combine the databases it receives from its various partners into a single, high-quality database with reasonable error margins.
This methodological approach is based on:
1. Data collection, processing and storage
From the reception of data through the development of indicators, the creation of the consolidated database follows a step-by-step iterative process.
This process begins with data scoping and requirements specification, performed in partnership with each data provider, to comprehend the data and specify the populations being investigated, as well as ensure a minimum level of database consistency.
When databases are received, qualitative studies are undertaken to assess the data's quality and potential (filling rate of identifiers and other fields, duplicate rate, etc.). These studies also enable the definition of the pre-processing to be performed on these databases (treatment of duplicates, creation of new fields and normalization of fields).
On the basis of an evaluation of the completion rates of each of the available identifiers (Common Business Identifier ICE, Tax Identifier, RC/Court Code, and CNSS No.), a cross-referencing process employing these identifiers is used to ensure that the merging of the databases is performed optimally and reliably, without information loss or the presence of duplicates. Other treatments, such as the application of management rules and data enrichment, complete it.
In the aftermath of this process, centralized databases are created, adhering to the principles governing the constitution of databases and the production of indicators, namely the uniqueness of a company's representation in these databases, its active nature, and its description with the highest quality information possible.
2. Analysis and dissemination:
A quality analysis is performed to determine the calculation perimeters once the Consolidated Base has been built and is ready to be used in the calculation of indicators. To that end, a repository is created, defining in detail the indicators, their calculation methods, their interpretation as well as their scope of study.
Once the indicators have been produced, they are framed by macroeconomic variables and compared with national and international trends. As part of the process of enriching and updating the list of indicators to be produced, the Observatory routinely monitors national and international studies and publications.
3. Data reliability strategy
The Observatory uses a five-tier strategy to ensure the accuracy of its data. It relies on multi-year data qualification processes revolving around the identification and reliability of the population of companies. This allows to use of the most qualified information available in each database, to complete missing information as much as possible as well as eliminating biases in the calculations related to the presence of duplicates.
Challenges encountered
At the level of data collection and processing, the Observatory has identified and dealt with several technical difficulties related to
- The absence of a common identification key, which has led to complexity in the cross-referencing and merging of databases, given that the Common Enterprise Identifier (CEI) is currently being implemented.
- The structure of the databases received, which are intended for the specific missions of each organization, does not allow them to be used for decision-making analyses.
- The use of non-standardized reference systems specific to each institution.
- The use of different nomenclatures in the coding of business activities.
- The unreliability of certain fields, the multitude of missing data and the redundancy of data.
Moreover, access to company data remains relatively limited. Together with its partners, the Observatory advocates for a greater decompartmentalization of government data, while maintaining the privacy of personal information and adhering to all applicable data processing regulations.
In fact, the decompartmentalization of government data is a guarantee of transparency that has a beneficial effect on international competitiveness rankings and enables countries to earn more GDP points. Indeed, data is viewed as a vector for improved public knowledge, which adds to an improved national business climate.
Technical and Scientific Framework
Data science as a lever of innovation for the development of SMEs
The use of firm data is a crucial component of SME development strategies. Indeed, "Big Data," artificial intelligence, the reconfiguration of value chains, strategies and organizations based on a "culture of data," and transparency, characterize the current forms of the digital revolution and enable companies to further exploit their growth and innovation potential.
The digital revolution facilitates the production, dissemination, and processing of mass data. The opening and sharing of this data have become a powerful lever to:
- strengthening the confidence of entrepreneurs and investors through greater transparency of public action.
- enable and support the creation of innovative business support services.
- improve the efficiency of government support programs for businesses and build useful tools to manage these programs.
- Support economic dynamism by creating new resources for innovation and growth.
- Create a dynamic for identifying data and structuring the ecosystem for its reuse in analysis and research (administration, researchers, academics, startups, associations, etc.)
In order to benefit from this rapid transformation, the Observatory intends to strengthen its cooperation with the academic and research world and is considering the creation of a college of experts in the field of technology, data science specialists, lawyers, economists, etc.
Data Science techniques used by the Observatory
The Observatory has established Data Science as the main lever of its action and its missions, namely the production of indicators and statistics with the best possible level of reliability and with acceptable margins of error.
Among the Data Science techniques used by the Observatory, we find:
Machine Learning: which uses statistical algorithms or neural networks. The performance of a Machine Learning algorithm is improved as it is trained on the data it processes, which is possible thanks to the enormous progress in computing and storage capacities.
Text Mining: which consists of analyzing sets of textual documents in order to capture key concepts and themes, and to identify hidden relationships and trends. It allows to transform text into structured data. Text mining automatically classifies texts by sentiment, subject or intent.
Web Scraping: involves extracting data from Internet sites and then recording and analyzing it. Scraping can be manual or automatic: the former refers to the fact of manually copying and inserting information and data, while the latter uses software or an algorithm that explores several websites in order to extract the desired information. In the case of the Observatory, the extracted data is public and freely accessible by third parties on the web.
Setting up the foundations for industrialization and automation of production
In 2020, the Observatory laid the foundations of the decision-making information system designed to strengthen its technical capacities. Indeed, the DIS will provide the Observatory with the technological foundations necessary for the industrialization and future development of its activities, by setting up a "data factory" for the automation of the entire value chain, from data reception to visualization and use.